Karton is a robust framework for creating flexible and lightweight malware analysis backends. It can be used to connect malware* analysis systems into a robust pipeline with very little effort.
We’ve been in the automation business for a long time. We’re dealing with more and more threats, and we have to automate everything to keep up with incidents. Because of this, we often end up with many scripts stuck together with duck duct tape and WD-40. These scripts are written by analysts in the heat of the moment, fragile and ugly – but they work, and produce intel that must be stored, processed further, sent to other systems or shared with other organisations.
We needed a way to take our PoC scripts and easily insert them into our analysis pipeline. We also wanted to monitor their execution, centralise logging, improve robustness, reduce development inertia… For this exact purpose, we created Karton.
* while Karton was designed with malware analysis in mind, it works nicely in every microservice-oriented project.
Installation
Installation is as easy as a single pip install
command:
pip3 install karton-core
In order to setup the whole backend environment you will also need MinIO and Redis, see the docs for details.
Getting Started
Installation
You can get the karton framework from pip:
python -m pip install karton-core
Or, if you’re feeling adventurous, download the sources using git and install them manually.
In addition to karton, you’ll also need to setup MinIO and Redis-server.
Configuration
Each Karton subsystem needs a karton.ini
file that contains the connection parameters for Redis and MinIO.
You can also use this file to store custom fields and use them e.g. by Overriding Config.
By default, the config class will look for the config file in several places, but let’s start by placing one in the root of our new karton subsystem.
[minio]
secret_key = minioadmin
access_key = minioadmin
address = localhost:9000
bucket = karton
secure = 0
[redis]
host=localhost
port=6379
If everything was configured correctly, you should now be able to run the karton-system
broker and get "Manager karton.system started"
signaling that it was able to connect to Redis and MinIO correctly.
Docker-compose Development Setup
If you’re just trying Karton out or you want a quick & easy development environment setup check out the dev
folder in the karton root directory.
It contains a small docker-compose setup that will setup the minimal development environment for you.
All you have to do is run
docker-compose up –build
And then connect additional karton systems using the karton.ini.dev
config file.
Writing Your First Producer And Consumer
Since all great examples start with foobar, that’s exactly what we’re going to do. Let’s start by writing a producer that spawns new tasks.
from karton.core import Producer, Task
if name == “main”:
foo_producer = Producer(identity=”foobar-producer”)
for i in range(5):
task = Task(headers={“type”: “foobar”}, payload={“data”: i})
foo_producer.send_task(task)
That was pretty short! Now for a bit longer consumer:
from karton.core import Consumer, Task
class FooBarConsumer(Consumer):
identity = “foobar-consumer”
filters = [
{
“type”: “foobar”
}
]
def process(self, task: Task) -> None:
num = task.get_payload(“data”)
print(num)
if num % 3 == 0:
print(“Foo”)
if num % 5 == 0:
print(“Bar”)
if name == “main”:
FooBarConsumer().loop()
If we now run the consumer and spawn a few “foobar” tasks we should get a few foobars logs in return:
[INFO] Service foo-consumer started
[INFO] Service binds created.
[INFO] Binding on: {‘type’: ‘foobar’}
[INFO] Received new task – 884880e0-e5fc-4a71-a93a-08f0caa92889
0
Foo
Bar
[INFO] Task done – 884880e0-e5fc-4a71-a93a-08f0caa92889
[INFO] Received new task – 60be2eb5-9e7e-4928-8823-a0d30bbe68ec
1
[INFO] Task done – 60be2eb5-9e7e-4928-8823-a0d30bbe68ec
[INFO] Received new task – 301d8a50-f21e-4e33-b30e-0f3b1cdbda03
2
[INFO] Task done – 301d8a50-f21e-4e33-b30e-0f3b1cdbda03
[INFO] Received new task – 3bb9aea2-4027-440a-8c21-57b6f476233a
3
Foo
[INFO] Task done – 3bb9aea2-4027-440a-8c21-57b6f476233a
[INFO] Received new task – 050cdace-05b0-4648-a070-bc4a7a8de702
4
[INFO] Task done – 050cdace-05b0-4648-a070-bc4a7a8de702
[INFO] Received new task – d3a39940-d64c-4033-a7da-80eae9786631
5
Bar
[INFO] Task done – d3a39940-d64c-4033-a7da-80eae9786631
Examples
Here are few examples of common Karton system patterns.
Producer
import sys
from karton.core import Config, Producer, Task, Resource
config = Config(“karton.ini”)
producer = Producer(config)
filename = sys.argv[1]
with open(filename, “rb”) as f:
contents = f.read()
resource = Resource(os.path.basename(filename), contents)
task = Task({“type”: “sample”, “kind”: “raw”})
task.add_resource(“sample”, resource)
task.add_payload(“tags”, [“simple_producer”])
task.add_payload(“additional_info”, [“This sample has been added by simple producer example”])
logging.info(‘pushing file to karton %s, task %s’ % (name, task))
producer.send_task(task)
Consumer
Consumer has to define identity
, a name used for identification and binding in RMQ and filters
– a list of dicts determining what types of tasks the service wants to process.
Elements in the list are OR’ed and items inside dicts are AND’ed.
import sys
from karton.core import Config, Consumer, Task, Resource
class Reporter(Consumer):
identity = “karton.reporter”
filters = [
{
“type”: “sample”,
“stage”: “recognized”
},
{
“type”: “sample”,
“stage”: “analyzed”
},
{
“type”: “config”
}
]
Above example accepts headers like:
{
“type”: “sample”,
“stage”: “recognized”,
“kind”: “runnable”,
“platform”: “win32”,
“extension”: “jar”
}
or
{
“type”: “config”,
“kind”: “cuckoo1”
}
but not
{
“type”: “sample”,
“stage”: “something”
}
Next step is to define process method, this is handler for incoming tasks that match our filters.
def process(self, task: Task) -> None:
if task.headers[“type”] == “sample”:
return self.process_sample(task)
else:
return self.process_config(task)
def process_sample(self, task: Task) -> None:
sample = task.get_resource(“sample”)
# …
def process_config(self, task: Task) -> None:
config = task.get_payload(“config”)
# …
task.headers
gives you information on why task was routed and methods like get_resource or get_payload allow you to get resources or metadata from task.
Finally, we need to run our module, we get this done with loop method, which blocks on listening for new tasks, running process when needed.
if name == “main”:
c = Reporter()
c.loop()
Karton
Karton class is simply Producer and Consumer bundled together.
As defined in karton/core/karton.py:
class Karton(Consumer, Producer):
“””
This glues together Consumer and Producer – which is the most common use case
“””
Receiving data is done exactly like in Consumer. Using producer is no different as well, just use self.send_task
.
Full-blown example below.
from karton.core import Karton, Task
class SomeNameKarton(Karton):
# Define identity and filters as you would in the Consumer class
identity = “karton.somename”
filters = [
{
“type”: “config”,
},
{
“type”: “analysis”,
“kind”: “cuckoo1”
},
]
# Method called by Karton library
def process(self, task: Task) -> None:
# Getting resources we need without downloading them locally
analysis_resource = task.get_resource(‘analysis’)
config_resource = task.get_resource(‘config’)
# Log with self.log
self.log.info(“Got resources, lets analyze them!”)
…
# Send our results for further processing or reporting
# Producer part
t = Task({“type”: “sample”})
t.add_resource(“sample”, Resource(filename, content))
self.send_task(task)
Overriding Config
Popular use case is to have another custom configuration in addition to the one needed for karton to work.
This can be easily done by overriding Config class and using that for Karton initialization.
import mwdblib
class MWDBConfig(Config):
def init(self, path=None) -> None:
super().init(path)
self.mwdb_config = dict(self.config.items(“mwdb”))
def mwdb(self) -> mwdblib.MWDB:
api_key=self.mwdb_config.get(“api_key”)
api_url=self.mwdb_config.get(“api_url”, mwdblib.api.API_URL)
mwdb = mwdblib.MWDB(api_key=api_key, api_url=api_url)
if not api_key:
mwdb.login(
self.mwdb_config[“username”],
self.mwdb_config[“password”])
return mwdb
Log consumer
By default, all logs created in Karton systems are published to a specialized log consumer using the Redis PUBSUB
pattern.
This is a very simple example of a system that implements the LogConsumer
interface and prints logs to stderr.
import sys
from karton.core.karton import LogConsumer
class StdoutLogger(LogConsumer):
identity = “karton.stdout-logger”
def process_log(self, event: dict) -> None:
# there are “log” and “operation” events
if event.get(“type”) == “log”:
print(f”{event[‘name’]}: {event[‘message’]}”, file=sys.stderr, flush=True)
if name == “main”:
StdoutLogger().loop()
Headers, Payloads And Resources
Task consists of two elements: headers and payload.
Task headers
Headers specify the purpose of a task and determine how task will be routed by karton-system. They’re defined by flat collection of keys and values.
Example:
task = Task(
headers = {
“type”: “sample”,
“kind”: “runnable”,
“platform”: “win32”,
“extension”: “dll”
}
)
Consumers listen for specific set of headers, which is defined by filters.
class GenericUnpacker(Karton):
“””
Performs sample unpacking
“””
identity = “karton.generic-unpacker”
filters = [
{
“type”: “sample”,
“kind”: “runnable”
},
{
“type”: “sample”,
“kind”: “script”,
“platform”: “win32”
}
]
def process(self, task: Task) -> None:
# Get incoming task headers
headers = task.headers
self.log.info(“Got %s sample from %s”, headers[“kind”], headers[“origin”])
If Karton-System finds that a task matches any of subsets defined by consumer queue filters then the task will be routed to that queue.
Following the convention proposed in examples above, it means that GenericUnpacker
will get all tasks contain samples directly runnable in sandboxes (regardless of target platform) or Windows 32-bit only scripts.
Headers can be used to process our input differently, depending on the kind of sample:
class GenericUnpacker(Karton):
…
def process(self, task: Task) -> None:
# Get incoming task headers
headers = task.headers
if headers[“kind”] == “runnable”:
self.process_runnable()
elif headers[“kind”] == “script”:
self.process_script()
Few headers have special meaning and are added automatically by Karton to incoming/outgoing tasks.
{"origin": "<identity>"}
specifies the identity of task sender. It can be used for listening for tasks incoming only from predefined identity.{"receiver": "<identity>"}
is added by Karton when task is routed to the consumer queue. On the receiver side, value is always equal toself.identity
Task payload
Payload is also a dictionary, but it’s not required to be a flat structure like headers are. Its contents do not affect the routing so task semantics must be defined by headers.
task = Task(
headers = …,
payload = {
“entrypoints”: [
“_ExampleFunction@12”
],
“matched_rules”: {
…
},
“sample”: Resource(“original_name.dll”, path=”uploads/original_name.dll”)
}
)
Payload can be accessed by Consumer using Task.get_payload()
method
class KartonService(Karton):
…
def process(self, task: Task) -> None:
entrypoints = task.get_payload(“entrypoints”, default=[])
But payload dictionary itself still must be lightweight and JSON-encodable, because it’s stored in Redis along with the whole task definition.
If task operates on binary blob or complex structure, which is probably the most common use-case, payload can still be used to store the reference to that object. The only requirement is that object must be placed in separate, shared storage, available for both Producer and Consumer. That’s exactly how Resource
objects work.
Resource Objects
Resources are part of a payload that represent a reference to the file or other binary large object. All objects of that kind are stored in MinIO, which is used as shared object storage between Karton subsystems.
task = Task(
headers = …,
payload = {
“sample”: Resource(“original_name.dll”, path=”uploads/original_name.dll”)
}
)
Resource objects created by producer (LocalResource
) are uploaded to MinIO and transformed to RemoteResource
objects. RemoteResource is lazy object that allows to download the object contents via RemoteResource.content
property.
class GenericUnpacker(Karton):
…
def unpack(self, packed_content: bytes) -> bytes:
…
def process(self, task: Task) -> None:
# Get sample resource
sample = task.get_resource(“sample”)
# Do the job
unpacked = self.unpack(sample.content)
# Publish the results
task = Task(
headers={
“type”: “sample”,
“kind”: “unpacked”
},
payload={
“sample”: Resource(“unpacked”, content=unpacked)
}
)
self.send_task(task)
If expected resource is too big for in-memory processing or we want to launch external tools that need the file system path, resource contents can be downloaded using RemoteResource.download_to_file()
or RemoteResource.download_temporary_file()
.
class KartonService(Karton):
…
def process(self, task: Task) -> None:
archive = task.get_resource(“archive”)
with archive.download_temporary_file() as f:
# f is file-like named object
archive_path = f.name
If you want to pass original sample along with new task, you can just put a reference back into its payload.
task = Task(
headers={
“type”: “sample”,
“kind”: “unpacked”
},
payload={
“sample”: Resource(“unpacked”, content=unpacked),
“parent”: sample # Reference to original (packed) sample
}
)
self.send_task(task)
Each resource has its own metadata store where we can provide additional information about file e.g. SHA-256 checksum
sample = Resource(“sample.exe”,
content=sample_content,
metadata={
“sha256”: hashlib.sha256(sample_content).hexdigest()
})
More information about resources can be found in API documentation.
Directory Resource Objects
Resource objects work well for single files, but sometimes we need to deal with bunch of artifacts e.g. process memory dumps from dynamic analysis. Very common way to do that is to pack them into Zip archive using Python zipfile module facilities.
Karton library includes a helper method for that kind of archives, called LocalResource.from_directory()
.
task = Task(
headers={
“type”: “analysis”
},
payload={
“dumps”: LocalResource.from_directory(analysis_id,
directory_path=f”analyses/{analysis_id}/dumps”),
}
)
self.send_task(task)
Files contained in directory_path
are stored under relative paths to the provided directory path. Default compression level is zipfile.ZIP_DEFLATED
instead of zipfile.ZIP_STORED
.
Directory resources are deserialized to the usual RemoteResource
objects but in contrary to the usual resources they can for example be extracted to directories using RemoteResource.extract_temporary()
class KartonService(Karton):
…
def process(self, task: Task) -> None:
dumps = task.get_resource(“dumps”)
with dumps.extract_temporary() as dumps_path:
…
If we don’t want to extract all files, we can work directly with zipfile.ZipFile
object, which will be internally downloaded from MinIO to the temporary file using RemoteResource.download_temporary_file()
method.
class KartonService(Karton):
…
def process(self, task: Task) -> None:
dumps = task.get_resource(“dumps”)
with dumps.zip_file() as zipf:
with zipf.open(“sample_info.txt”) as info:
…
More information about resources can be found in API documentation.
Persistent Payload
Part of payload that is propagated to the whole task subtree. The common use-case is to keep information related not with single artifact but the whole analysis, so they’re available everywhere even if not explicitly passed by the Karton Service.
task = Task(
headers=…,
payload=…,
payload_persistent={
“uploader”: “psrok1”
}
)
Incoming persistent payload (task received by Karton Service) is merged by Karton library with the outgoing tasks (result tasks sent by Karton Service). Karton service can’t overwrite or delete the incoming payload keys.
class KartonService(Karton):
…
def process(self, task: Task) -> None:
uploader = task.get_payload(“uploader”)
assert task.is_payload_persistent(“uploader”)
task = Task(
headers=…,
payload=…
)
# Outgoing task also contains “uploader” key
self.send_task(task)
Regular payloads and persistent payload keys have common namespace so persistent payload can’t be overwritten by regular payload as well e.g.
task = Task(
headers=…,
payload={
“common_key”: “”
},
payload_persistent={
“common_key”: “”
}
)
Advanced Concepts
Routed and unrouted tasks (task forking)
During its lifetime, the task will transfer between various states and its reference will be passed through several queues, a simple way to understand it is to see how the tasks state changes in various moments:
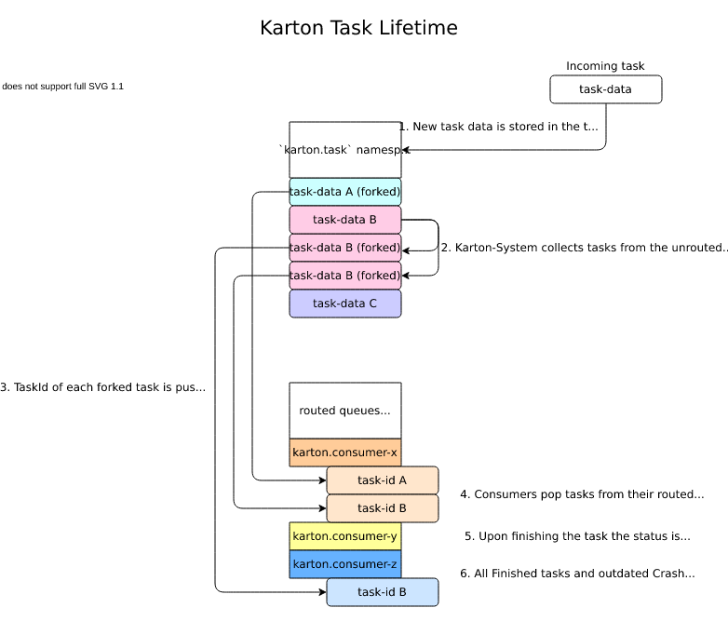
Each new task is registered in the system by a call to karton.Producer.send_task()
and starts its life in the unrouted task queue with a TaskState.Declared
state.
All actual task data is stored in the Karton.task
namespace and all other (routed and unrouted) queues will be always only holding a reference to a record from this place.
The main broker – karton.System
constantly looks over the unrouted (karton.tasks
) queue and keeps the tasks running as well as clears up leftover unneeded data.
Because task headers can be accepted by more than one consumer the task has to be forked before it goes to the appropriate consumer (routed) queues. Based on unrouted task, Karton.System
generates as many routed tasks as there are matching queues. These tasks are separate, independent instances, so they have different uid than original unrouted task.
Note
While uid of routed and unrouted tasks are different, parent_uid stays the same. parent_uid always identifies the routed task.
Reference to the unrouted task is called orig_uid.
Each registered consumer monitors its (routed) queue and performs analysis on all tasks that appear there. As soon as the consumer starts working on a given task, it sends a signal to the broker to mark the tasks state as TaskState.Started
.
If everything goes smoothly, the consumer finishes the tasks and sends a similar signal, this time marking the task as TaskState.Finished
. If there is a problem and an exception is thrown within the self.process
function, TaskState.Crashed
is used instead.
As a part of its housekeeping, Karton.System
removes all TaskState.Finished
tasks immediately and TaskState.Crashed
tasks after a certain grace period to allow for inspection and optional retry.
Task tree (analysis) and task life cycle
Every analysis starts from initial task spawned by karton.Producer
. Initial task is consumed by consumers, which then produce next tasks for further processing. These various tasks originating from initial task can be grouped together into a task tree, representing the analysis
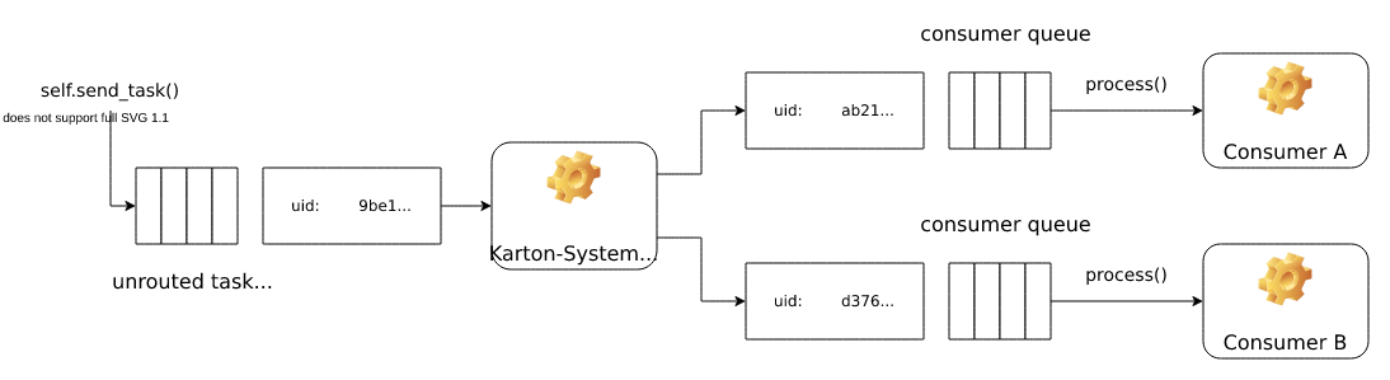
Each task is identified by a tuple of four identifiers:
- uid – unique task identifier
- parent_uid – identifier of task that spawned current task as a result of processing
- root_uid – task tree identifier (analysis identifier, derived from uid of initial unrouted task)
- orig_uid – identifier of the original task that was forked to create this task (unrouted task or retried crashed task)
In order to better understand how those identifiers are inherited and passed between tasks take a look at the following example:
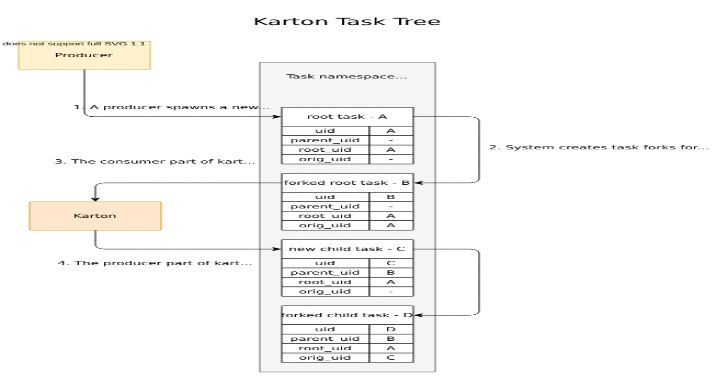
Handling logging
By default, all systems inheriting from karton.core.KartonBase()
will have a custom logging.Logger()
instance exposed as log()
. It publishes all logged messages to a special PUBSUB key on the central Redis database.
In order to store the logs into a persistent storage like Splunk or Rsyslog you have to implement a service that will consume the log entries and send them to the final database, for an example of such service see Log consumer.
The logging level can be configured using the standard karton config and setting level
in the logging
section to appropriate level like "DEBUG"
, "INFO"
or "ERROR"
.
Consumer queue persistence
Consumer queue is created on the first registration of consumer and it gets new tasks even if all consumer instances are offline. It guarantees that analysis will complete even after short downtime of part of subsystems. Unfortunately, it also blocks completion of the analysis when we connect a Karton Service which is currently developed or temporary.
We can turn off queue persistence using the persistent = False
attribute in the Karton subsystem class.
class TemporaryConsumer(Karton):
identity = “karton.temporary-consumer”
filters = …
persistent = False
def process(self, task: Task) -> None:
…
This is also the (hacky) way to remove persistent queue from the system. Just launch empty consumer with identity you want to remove, wait until all tasks will be consumed and shut down the consumer.
from karton.core import Karton
class DeleteThisConsumer(Karton):
identity = “karton.identity-to-be-removed”
filters = {}
persistent = False
def process(self, task: Task) -> None:
pass
DeleteThisConsumer().loop()
Prioritized tasks
Karton allows to set priority for task tree: TaskPriority.HIGH
, TaskPriority.NORMAL
(default) or TaskPriority.LOW
. Priority is determined by producer spawning an initial task.
producer = Producer()
task = Task(
headers=…,
priority=TaskPriority.HIGH
)
producer.send_task(task)
All tasks within the same task tree have the same priority, which is derived from the priority of initial task. If consumer will try to set different priority for spawned tasks, new priority settings will be simply ignored.
Extending configuration
During processing we may need to fetch data from external service or use libraries that need to be pre-configured. The simplest approach is to use separate configuration file, but this is a bit messy.
Karton configuration is represented by special object karton.Config
, which can be explicitly provided as an argument to the Karton constructor. Config is based on configparser.ConfigParser
, so we can extend it with additional sections for custom configuration.
For example, if we need to communicate with MWDB, we can make MWDB binding available via self.config.mwdb
import mwdblib
class MWDBConfig(Config):
def init(self, path=None) -> None:
super().init(path)
self.mwdb_config = dict(self.config.items(“mwdb”))
def mwdb(self) -> mwdblib.MWDB:
api_key=self.mwdb_config.get(“api_key”)
api_url=self.mwdb_config.get(“api_url”, mwdblib.api.API_URL)
mwdb = mwdblib.MWDB(api_key=api_key, api_url=api_url)
if not api_key:
mwdb.login(
self.mwdb_config[“username”],
self.mwdb_config[“password”])
return mwdb
class GenericUnpacker(Karton):
…
def process(self, task: Task) -> None:
file_hash = task.get_payload(“file_hash”)
sample = self.config.mwdb().query_file(file_hash)
if name == “main”:
GenericUnpacker(MWDBConfig()).loop()
and provide additional section in karton.ini file:
[minio]
secret_key =
access_key =
address = 127.0.0.1:9000
bucket = karton
secure = 0
[redis]
host = 127.0.0.1
port = 6379
[mwdb]
api_url = http://127.0.0.1:5000/api
api_key =
Karton-wide and instance-wide configuration
By default the configuration is searched in the following locations (by searching order):
/etc/karton/karton.ini
~/.config/karton/karton.ini
./karton.ini
- environment variables
Each next level overrides and merges with the values loaded from the previous path. It means that we can provide karton-wide configuration and specialized instance-wide extended configuration specific for subsystem.
Contents of /etc/karton/karton.ini
:
[minio]
secret_key =
access_key =
address = 127.0.0.1:9000
bucket = karton
secure = 0
[redis]
host = 127.0.0.1
port = 6379
and specialized configuration in the working directory ./karton.ini
[mwdb]
api_url = http://127.0.0.1:5000/api
api_key =
Passing Tasks To The External Queue
Karton can be used to delegate tasks to separate queues e.g. external sandbox. External sandboxes usually have their own concurrency and queueing mechanisms, so Karton subsystem needs to:
- dispatch task to the external service
- wait until service ends processing
- fetch results and spawn result tasks keeping the root_uid and parent_uid
We tried to solve this using asynchronous tasks but it turned out to be very hard to be implemented correctly and didn’t really fit in to with the Karton model.
Busy waiting
The simplest way to do that is to perform all of these actions synchronously, inside the process()
method.
def process(self, task: Task) -> None:
sample = task.get_resource(“sample”)
#Dispatch task, getting the analysis_id
with sample.download_temporary_file() as f:
analysis_id = sandbox.push_file(f)
#Wait until analysis finish
while sandbox.is_finished(analysis_id):
# Check every 5 seconds
time.sleep(5)
#If analysis has been finished: get the results and process them
analysis = sandbox.get_results(analysis_id)
self.process_results(analysis)
Writing Unit Tests
Basic unit test
So you want to test your karton systems, that’s great! The karton core actually comes with a few helper methods to make it a bit easier.
The building block of all karton tests is karton.core.test.KartonTestCase()
. It’s a nifty class that wraps around your karton system and allows you to run tasks on it without needing to create a producer. What’s more important however, is that it runs without any Redis or MinIO interaction and thus creates no side effects.
from math_karton import MathKarton
from karton.core.test import KartonTestCase
class MathKartonTestCase(KartonTestCase):
“””Test a karton that accepts an array of integers in “numbers” payload and
returns their sum in “result”.
“””
karton_class = MathKarton
def test_addition(self) -> None:
# prepare a fake test task that matches the production format
task = Task({
“type”: “math-task”,
}, payload={
“numbers”: [1, 2, 3, 4],
})
# dry-run the fake task on the wrapped karton system
results = self.run_task(task)
# prepare a expected output task and check if it matches the one produced
expected_task = Task(
“type”: “math-result”
}, payload={
“result”: 10,
})
self.assertTasksEqual(results, expected_task)
Testing resources
That was pretty simple, but what about testing karton systems that accept and spawn payloads containing resources?
karton.core.test.KartonTestCase()
already takes care of them for you. Just use normal karton.core.Resource()
like you would normally do.
from reverse import ReverserKarton
from karton.core.test import KartonTestCase
from karton.core import Resource
class ReverserKartonTestCase(KartonTestCase):
“””Test a karton that expects a KartonResource in “file” key and spawns a new
task containing that file reversed.
“””
karton_class = ReverserKarton
def test_reverse(self) -> None:
# load data from testcase files
with open(“testdata/file.txt”, “rb”) as f:
input_data = f.read()
# create fake, mini-independent resources
input_sample = Resource(“sample.txt”, input_data)
output_sample = Resource(“sample.txt”, input_data[::-1])
# prepare a fake test task that matches the production format
task = Task({
“type”: “reverse-task”,
}, payload={
“file”: input_sample
})
# dry-run the fake task on the wrapped karton system
results = self.run_task(task)
# prepare a expected output task and check if it matches the one produced
expected_task = Task(
“type”: “reverse-result”
}, payload={
“file”: output_sample,
})
self.assertTasksEqual(results, expected_task)
Example Usage
To use karton you have to provide class that inherits from Karton.
from karton.core import Karton, Task, Resource
class GenericUnpacker(Karton):
“””
Performs sample unpacking
“””
identity = “karton.generic-unpacker”
filters = [
{
“type”: “sample”,
“kind”: “runnable”,
“platform”: “win32”
}
]
def process(self, task: Task) -> None:
# Get sample object
packed_sample = task.get_resource(‘sample’)
# Log with self.log
self.log.info(f”Hi {packed_sample.name}, let me analyze you!”)
…
# Send our results for further processing or reporting
task = Task(
{
“type”: “sample”,
“kind”: “raw”
}, payload = {
“parent”: packed_sample,
“sample”: Resource(filename, unpacked)
})
self.send_task(task)
if name == “main”:
# Here comes the main loop
GenericUnpacker().loop()
Karton Systems
Some Karton systems are universal and useful to everyone. We decided to share them with the community.
This repository. It contains the karton.system
service – main service, responsible for dispatching tasks within the system. It also contains the karton.core
module, that is used as a library by other systems.
A small Flask dashboard for task and queue management and monitoring.
The “router”. It recognises samples/files and produces various task types depending on the file format. Thanks to this, other systems may only listen for tasks with a specific format (for example, only zip
archives).
Generic archive unpacker. Archives uploaded into the system will be extracted, and every file will be processed individually.
Malware extractor. It uses Yara rules and Python modules to extract static configuration from malware samples and analyses. It’s a fishing rod, not a fish – we don’t share the modules themselves. But it’s easy to write your own!
A very important part of the pipeline. Reporter submits all files, tags, comments and other intel produced during the analysis to MWDB. If you don’t use MWDB yet or just prefer other backends, it’s easy to write your own reporter.
Automatically runs Yara rules on all files in the pipeline, and tags samples appropriately. Rules not included ;).
Karton system that decodes files encoded with common methods, like hex
, base64
, etc. (You wouldn’t believe how common it is).
A small wrapper around AutoIt-Ripper that extracts embedded AutoIt scripts and resources from compiled AutoIt executables.
Automated black-box malware analysis system with DRAKVUF engine under the hood, which does not require an agent on guest OS.
Coming soon:
karton-misp-pusher
A reporter, that submits observed events to MISP.
This is how these systems can be used to form a basic malware analysis pipeline:
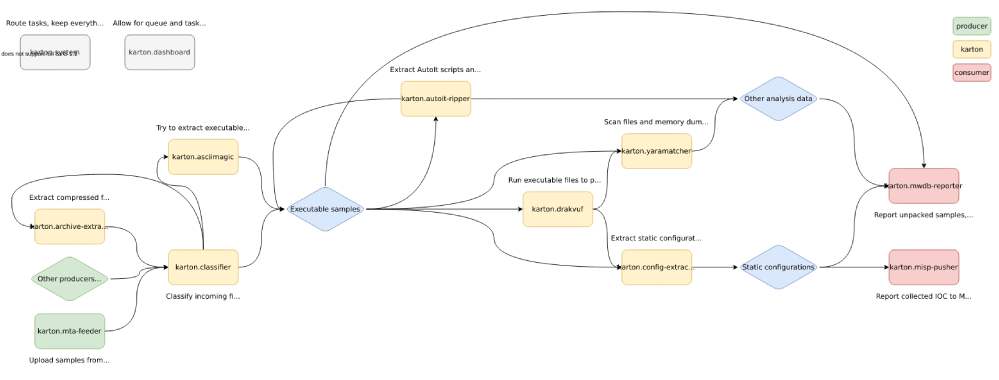